class: title .small[ Deploying and Scaling Microservices
with Docker and Kubernetes .small[.small[ **Be kind to the WiFi!** *Don't use your hotspot*
*Don't stream videos from YouTube, Netflix, etc.
(if you're bored, watch local content instead)* Thank you! ] ] ] .debug[(inline)] --- ## Intros - Hello! We are Jérôme ([@jpetazzo](https://twitter.com/jpetazzo), Docker Inc.) & AJ ([@s0ulshake](https://twitter.com/s0ulshake), Travis CI) -- - This is our first time doing this -- - But ... containers and us go back a long way -- 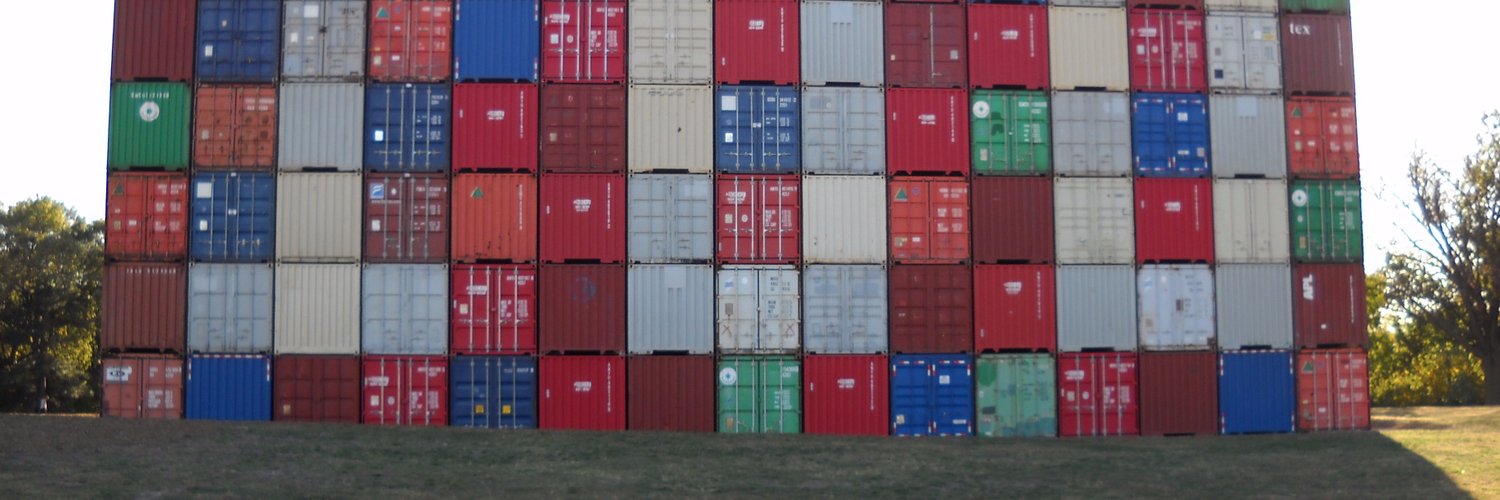 -- - In the immortal words of [Chelsea Manning](https://twitter.com/xychelsea): #WeGotThis! .debug[(inline)] --- ## Logistics - The tutorial will run from 9:00am to 12:15pm - There will be a coffee break at 10:30am
(please remind me if I forget about it!) - This will be fast-paced, but DON'T PANIC!
(all the content is publicly available) - Feel free to interrupt for questions at any time - Live feedback, questions, help on [Gitter](https://gitter.im/jpetazzo/workshop-20171026-prague) .debug[(inline)] --- ## About these slides - Your one-stop shop to awesomeness: http://container.training/ - The content that you're viewing right now is in a public GitHub repository: https://github.com/jpetazzo/orchestration-workshop - Typos? Mistakes? Questions? Feel free to hover over the bottom of the slide ... -- .footnote[👇 Try it! The source file will be shown and you can view it on GitHub and fork and edit it.] .debug[[kube/intro-ks.md](https://github.com/jpetazzo/container.training/tree/osseu17/slides/kube/intro-ks.md)] --- name: toc-chapter-1 ## Chapter 1 - [Pre-requirements](#toc-pre-requirements) - [Our sample application](#toc-our-sample-application) - [Running the application](#toc-running-the-application) - [Identifying bottlenecks](#toc-identifying-bottlenecks) .debug[(auto-generated TOC)] --- name: toc-chapter-2 ## Chapter 2 - [Kubernetes concepts](#toc-kubernetes-concepts) - [Declarative vs imperative](#toc-declarative-vs-imperative) - [Kubernetes network model](#toc-kubernetes-network-model) - [First contact with `kubectl`](#toc-first-contact-with-kubectl) - [Setting up Kubernetes](#toc-setting-up-kubernetes) - [Running our first containers on Kubernetes](#toc-running-our-first-containers-on-kubernetes) .debug[(auto-generated TOC)] --- name: toc-chapter-3 ## Chapter 3 - [Exposing containers](#toc-exposing-containers) - [Deploying a self-hosted registry](#toc-deploying-a-self-hosted-registry) - [Exposing services internally ](#toc-exposing-services-internally-) - [Exposing services for external access](#toc-exposing-services-for-external-access) - [The Kubernetes dashboard](#toc-the-kubernetes-dashboard) - [Security implications of `kubectl apply`](#toc-security-implications-of-kubectl-apply) .debug[(auto-generated TOC)] --- name: toc-chapter-4 ## Chapter 4 - [Scaling a deployment](#toc-scaling-a-deployment) - [Daemon sets](#toc-daemon-sets) - [Updating a service through labels and selectors](#toc-updating-a-service-through-labels-and-selectors) - [Rolling updates](#toc-rolling-updates) - [Next steps](#toc-next-steps) .debug[(inline)] --- name: toc-pre-requirements class: title Pre-requirements .nav[[Back to table of contents](#toc-chapter-1)] .debug[(automatically generated title slide)] --- # Pre-requirements - Computer with internet connection and a web browser - For instructor-led workshops: an SSH client to connect to remote machines - on Linux, OS X, FreeBSD... you are probably all set - on Windows, get [putty](http://www.putty.org/), Microsoft [Win32 OpenSSH](https://github.com/PowerShell/Win32-OpenSSH/wiki/Install-Win32-OpenSSH), [Git BASH](https://git-for-windows.github.io/), or [MobaXterm](http://mobaxterm.mobatek.net/) - A tiny little bit of Docker knowledge (that's totally OK if you're not a Docker expert!) .debug[[kube/prereqs-k8s.md](https://github.com/jpetazzo/container.training/tree/osseu17/slides/kube/prereqs-k8s.md)] --- class: in-person, extra-details ## Nice-to-haves - [Mosh](https://mosh.org/) instead of SSH, if your internet connection tends to lose packets
(available with `(apt|yum|brew) install mosh`; then connect with `mosh user@host`) - [GitHub](https://github.com/join) account
(if you want to fork the repo) - [Slack](https://community.docker.com/registrations/groups/4316) account
(to join the conversation after the workshop) - [Docker Hub](https://hub.docker.com) account
(it's one way to distribute images on your cluster) .debug[[kube/prereqs-k8s.md](https://github.com/jpetazzo/container.training/tree/osseu17/slides/kube/prereqs-k8s.md)] --- class: extra-details ## Extra details - This slide should have a little magnifying glass in the top left corner (If it doesn't, it's because CSS is hard — Jérôme is only a backend person, alas) - Slides with that magnifying glass indicate slides providing extra details - Feel free to skip them if you're in a hurry! .debug[[kube/prereqs-k8s.md](https://github.com/jpetazzo/container.training/tree/osseu17/slides/kube/prereqs-k8s.md)] --- ## Hands-on sections - The whole workshop is hands-on - We will see Docker and Kubernetes in action - You are invited to reproduce all the demos - All hands-on sections are clearly identified, like the gray rectangle below .exercise[ - This is the stuff you're supposed to do! - Go to [container.training](http://container.training/) to view these slides - Join the chat room on [Gitter](https://gitter.im/jpetazzo/workshop-20171026-prague) ] .debug[[kube/prereqs-k8s.md](https://github.com/jpetazzo/container.training/tree/osseu17/slides/kube/prereqs-k8s.md)] --- class: pic, in-person 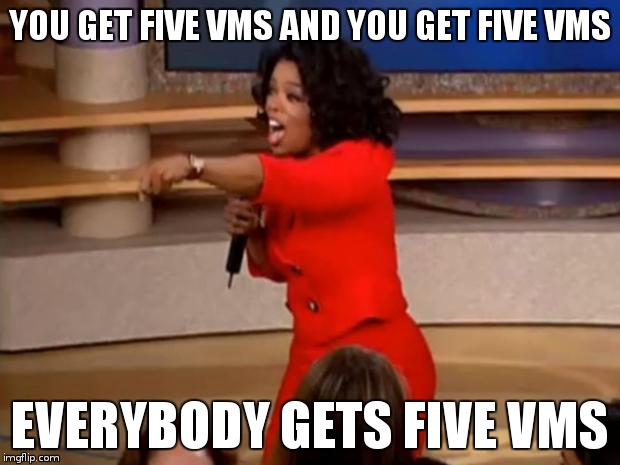 .debug[[kube/prereqs-k8s.md](https://github.com/jpetazzo/container.training/tree/osseu17/slides/kube/prereqs-k8s.md)] --- class: in-person ## You get five VMs - Each person gets 5 private VMs (not shared with anybody else) - Kubernetes has been deployed and pre-configured on these machines - They'll remain up until the day after the tutorial - You should have a little card with login+password+IP addresses - You can automatically SSH from one VM to another .exercise[ - Log into the first VM (`node1`) with SSH or MOSH - Check that you can SSH (without password) to `node2`: ```bash ssh node2 ``` - Type `exit` or `^D` to come back to node1 ] .debug[[kube/prereqs-k8s.md](https://github.com/jpetazzo/container.training/tree/osseu17/slides/kube/prereqs-k8s.md)] --- ## We will (mostly) interact with node1 only - Unless instructed, **all commands must be run from the first VM, `node1`** - We will only checkout/copy the code on `node1` - During normal operations, we do not need access to the other nodes - If we had to troubleshoot issues, we would use a combination of: - SSH (to access system logs, daemon status...) - Docker API (to check running containers and container engine status) .debug[[kube/prereqs-k8s.md](https://github.com/jpetazzo/container.training/tree/osseu17/slides/kube/prereqs-k8s.md)] --- ## Terminals Once in a while, the instructions will say:
"Open a new terminal." There are multiple ways to do this: - create a new window or tab on your machine, and SSH into the VM; - use screen or tmux on the VM and open a new window from there. You are welcome to use the method that you feel the most comfortable with. .debug[[kube/prereqs-k8s.md](https://github.com/jpetazzo/container.training/tree/osseu17/slides/kube/prereqs-k8s.md)] --- ## Tmux cheatsheet - Ctrl-b c → creates a new window - Ctrl-b n → go to next window - Ctrl-b p → go to previous window - Ctrl-b " → split window top/bottom - Ctrl-b % → split window left/right - Ctrl-b Alt-1 → rearrange windows in columns - Ctrl-b Alt-2 → rearrange windows in rows - Ctrl-b arrows → navigate to other windows - Ctrl-b d → detach session - tmux attach → reattach to session .debug[[kube/prereqs-k8s.md](https://github.com/jpetazzo/container.training/tree/osseu17/slides/kube/prereqs-k8s.md)] --- ## Brand new versions! - Kubernetes 1.8 - Docker Engine 17.10 - Docker Compose 1.16 .exercise[ - Check all installed versions: ```bash kubectl version docker version docker-compose -v ``` ] .debug[[kube/versions-k8s.md](https://github.com/jpetazzo/container.training/tree/osseu17/slides/kube/versions-k8s.md)] --- class: extra-details ## Kubernetes and Docker compatibility - Kubernetes only validates Docker Engine versions 1.11.2, 1.12.6, 1.13.1, and 17.03.2 -- class: extra-details - Are we living dangerously? -- class: extra-details - "Validates" = continuous integration builds - The Docker API is versioned, and offers strong backward-compatibility (If a client uses e.g. API v1.25, the Docker Engine will keep behaving the same way) .debug[[kube/versions-k8s.md](https://github.com/jpetazzo/container.training/tree/osseu17/slides/kube/versions-k8s.md)] --- name: toc-our-sample-application class: title Our sample application .nav[[Back to table of contents](#toc-chapter-1)] .debug[(automatically generated title slide)] --- # Our sample application - Visit the GitHub repository with all the materials of this workshop:
https://github.com/jpetazzo/orchestration-workshop - The application is in the [dockercoins]( https://github.com/jpetazzo/orchestration-workshop/tree/master/dockercoins) subdirectory - Let's look at the general layout of the source code: there is a Compose file [docker-compose.yml]( https://github.com/jpetazzo/orchestration-workshop/blob/master/dockercoins/docker-compose.yml) ... ... and 4 other services, each in its own directory: - `rng` = web service generating random bytes - `hasher` = web service computing hash of POSTed data - `worker` = background process using `rng` and `hasher` - `webui` = web interface to watch progress .debug[[common/sampleapp.md](https://github.com/jpetazzo/container.training/tree/osseu17/slides/common/sampleapp.md)] --- class: extra-details ## Compose file format version *Particularly relevant if you have used Compose before...* - Compose 1.6 introduced support for a new Compose file format (aka "v2") - Services are no longer at the top level, but under a `services` section - There has to be a `version` key at the top level, with value `"2"` (as a string, not an integer) - Containers are placed on a dedicated network, making links unnecessary - There are other minor differences, but upgrade is easy and straightforward .debug[[common/sampleapp.md](https://github.com/jpetazzo/container.training/tree/osseu17/slides/common/sampleapp.md)] --- ## Links, naming, and service discovery - Containers can have network aliases (resolvable through DNS) - Compose file version 2+ makes each container reachable through its service name - Compose file version 1 did require "links" sections - Our code can connect to services using their short name (instead of e.g. IP address or FQDN) - Network aliases are automatically namespaced (i.e. you can have multiple apps declaring and using a service named `database`) .debug[[common/sampleapp.md](https://github.com/jpetazzo/container.training/tree/osseu17/slides/common/sampleapp.md)] --- ## Example in `worker/worker.py` 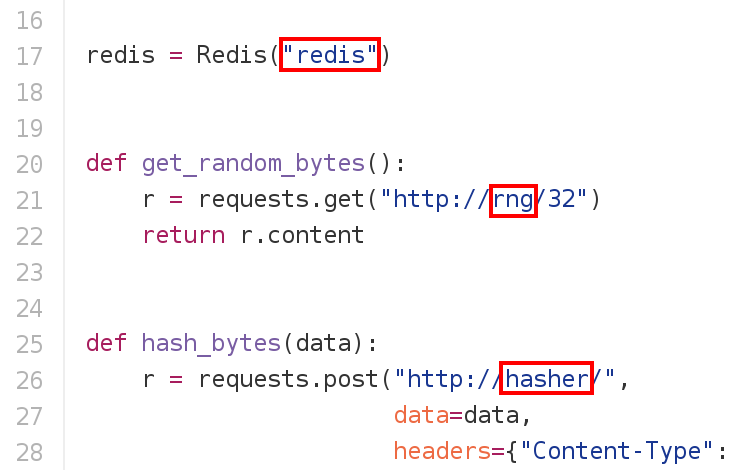 .debug[[common/sampleapp.md](https://github.com/jpetazzo/container.training/tree/osseu17/slides/common/sampleapp.md)] --- ## What's this application? .debug[[common/sampleapp.md](https://github.com/jpetazzo/container.training/tree/osseu17/slides/common/sampleapp.md)] --- class: pic 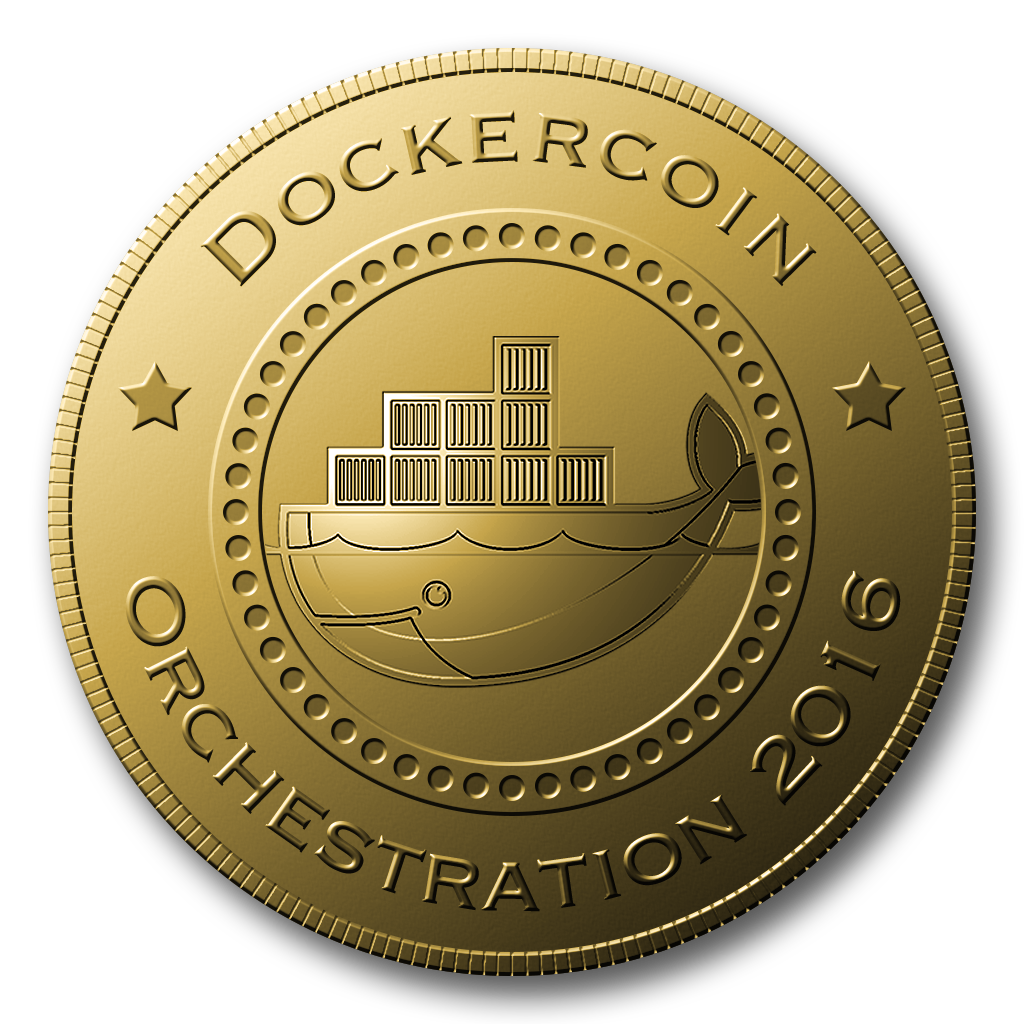 (DockerCoins 2016 logo courtesy of [@XtlCnslt](https://twitter.com/xtlcnslt) and [@ndeloof](https://twitter.com/ndeloof). Thanks!) .debug[[common/sampleapp.md](https://github.com/jpetazzo/container.training/tree/osseu17/slides/common/sampleapp.md)] --- ## What's this application? - It is a DockerCoin miner! 💰🐳📦🚢 -- - No, you can't buy coffee with DockerCoins -- - How DockerCoins works: - `worker` asks to `rng` to generate a few random bytes - `worker` feeds these bytes into `hasher` - and repeat forever! - every second, `worker` updates `redis` to indicate how many loops were done - `webui` queries `redis`, and computes and exposes "hashing speed" in your browser .debug[[common/sampleapp.md](https://github.com/jpetazzo/container.training/tree/osseu17/slides/common/sampleapp.md)] --- ## Getting the application source code - We will clone the GitHub repository - The repository also contains scripts and tools that we will use through the workshop .exercise[ - Clone the repository on `node1`: ```bash git clone git://github.com/jpetazzo/orchestration-workshop ``` ] (You can also fork the repository on GitHub and clone your fork if you prefer that.) .debug[[common/sampleapp.md](https://github.com/jpetazzo/container.training/tree/osseu17/slides/common/sampleapp.md)] --- name: toc-running-the-application class: title Running the application .nav[[Back to table of contents](#toc-chapter-1)] .debug[(automatically generated title slide)] --- # Running the application Without further ado, let's start our application. .exercise[ - Go to the `dockercoins` directory, in the cloned repo: ```bash cd ~/orchestration-workshop/dockercoins ``` - Use Compose to build and run all containers: ```bash docker-compose up ``` ] Compose tells Docker to build all container images (pulling the corresponding base images), then starts all containers, and displays aggregated logs. .debug[[common/sampleapp.md](https://github.com/jpetazzo/container.training/tree/osseu17/slides/common/sampleapp.md)] --- ## Lots of logs - The application continuously generates logs - We can see the `worker` service making requests to `rng` and `hasher` - Let's put that in the background .exercise[ - Stop the application by hitting `^C` ] - `^C` stops all containers by sending them the `TERM` signal - Some containers exit immediately, others take longer
(because they don't handle `SIGTERM` and end up being killed after a 10s timeout) .debug[[common/sampleapp.md](https://github.com/jpetazzo/container.training/tree/osseu17/slides/common/sampleapp.md)] --- ## Restarting in the background - Many flags and commands of Compose are modeled after those of `docker` .exercise[ - Start the app in the background with the `-d` option: ```bash docker-compose up -d ``` - Check that our app is running with the `ps` command: ```bash docker-compose ps ``` ] `docker-compose ps` also shows the ports exposed by the application. .debug[[common/sampleapp.md](https://github.com/jpetazzo/container.training/tree/osseu17/slides/common/sampleapp.md)] --- class: extra-details ## Viewing logs - The `docker-compose logs` command works like `docker logs` .exercise[ - View all logs since container creation and exit when done: ```bash docker-compose logs ``` - Stream container logs, starting at the last 10 lines for each container: ```bash docker-compose logs --tail 10 --follow ``` ] Tip: use `^S` and `^Q` to pause/resume log output. .debug[[common/sampleapp.md](https://github.com/jpetazzo/container.training/tree/osseu17/slides/common/sampleapp.md)] --- class: extra-details ## Upgrading from Compose 1.6 .warning[The `logs` command has changed between Compose 1.6 and 1.7!] - Up to 1.6 - `docker-compose logs` is the equivalent of `logs --follow` - `docker-compose logs` must be restarted if containers are added - Since 1.7 - `--follow` must be specified explicitly - new containers are automatically picked up by `docker-compose logs` .debug[[common/sampleapp.md](https://github.com/jpetazzo/container.training/tree/osseu17/slides/common/sampleapp.md)] --- ## Connecting to the web UI - The `webui` container exposes a web dashboard; let's view it .exercise[ - With a web browser, connect to `node1` on port 8000 - Remember: the `nodeX` aliases are valid only on the nodes themselves - In your browser, you need to enter the IP address of your node ] You should see a speed of approximately 4 hashes/second. More precisely: 4 hashes/second, with regular dips down to zero.
This is because Jérôme is incapable of writing good frontend code.
Don't ask. Seriously, don't ask. This is embarrassing. .debug[[common/sampleapp.md](https://github.com/jpetazzo/container.training/tree/osseu17/slides/common/sampleapp.md)] --- class: extra-details ## Why does the speed seem irregular? - The app actually has a constant, steady speed: 3.33 hashes/second
(which corresponds to 1 hash every 0.3 seconds, for *reasons*) - The worker doesn't update the counter after every loop, but up to once per second - The speed is computed by the browser, checking the counter about once per second - Between two consecutive updates, the counter will increase either by 4, or by 0 - The perceived speed will therefore be 4 - 4 - 4 - 0 - 4 - 4 - etc. *We told you to not ask!!!* .debug[[common/sampleapp.md](https://github.com/jpetazzo/container.training/tree/osseu17/slides/common/sampleapp.md)] --- ## Scaling up the application - Our goal is to make that performance graph go up (without changing a line of code!) -- - Before trying to scale the application, we'll figure out if we need more resources (CPU, RAM...) - For that, we will use good old UNIX tools on our Docker node .debug[[common/sampleapp.md](https://github.com/jpetazzo/container.training/tree/osseu17/slides/common/sampleapp.md)] --- ## Looking at resource usage - Let's look at CPU, memory, and I/O usage .exercise[ - run `top` to see CPU and memory usage (you should see idle cycles) - run `vmstat 1` to see I/O usage (si/so/bi/bo)
(the 4 numbers should be almost zero, except `bo` for logging) ] We have available resources. - Why? - How can we use them? .debug[[common/sampleapp.md](https://github.com/jpetazzo/container.training/tree/osseu17/slides/common/sampleapp.md)] --- ## Scaling workers on a single node - Docker Compose supports scaling - Let's scale `worker` and see what happens! .exercise[ - Start one more `worker` container: ```bash docker-compose scale worker=2 ``` - Look at the performance graph (it should show a x2 improvement) - Look at the aggregated logs of our containers (`worker_2` should show up) - Look at the impact on CPU load with e.g. top (it should be negligible) ] .debug[[common/sampleapp.md](https://github.com/jpetazzo/container.training/tree/osseu17/slides/common/sampleapp.md)] --- ## Adding more workers - Great, let's add more workers and call it a day, then! .exercise[ - Start eight more `worker` containers: ```bash docker-compose scale worker=10 ``` - Look at the performance graph: does it show a x10 improvement? - Look at the aggregated logs of our containers - Look at the impact on CPU load and memory usage ] .debug[[common/sampleapp.md](https://github.com/jpetazzo/container.training/tree/osseu17/slides/common/sampleapp.md)] --- name: toc-identifying-bottlenecks class: title Identifying bottlenecks .nav[[Back to table of contents](#toc-chapter-1)] .debug[(automatically generated title slide)] --- # Identifying bottlenecks - You should have seen a 3x speed bump (not 10x) - Adding workers didn't result in linear improvement - *Something else* is slowing us down -- - ... But what? -- - The code doesn't have instrumentation - Let's use state-of-the-art HTTP performance analysis!
(i.e. good old tools like `ab`, `httping`...) .debug[[common/sampleapp.md](https://github.com/jpetazzo/container.training/tree/osseu17/slides/common/sampleapp.md)] --- ## Accessing internal services - `rng` and `hasher` are exposed on ports 8001 and 8002 - This is declared in the Compose file: ```yaml ... rng: build: rng ports: - "8001:80" hasher: build: hasher ports: - "8002:80" ... ``` .debug[[common/sampleapp.md](https://github.com/jpetazzo/container.training/tree/osseu17/slides/common/sampleapp.md)] --- ## Measuring latency under load We will use `httping`. .exercise[ - Check the latency of `rng`: ```bash httping -c 10 localhost:8001 ``` - Check the latency of `hasher`: ```bash httping -c 10 localhost:8002 ``` ] `rng` has a much higher latency than `hasher`. .debug[[common/sampleapp.md](https://github.com/jpetazzo/container.training/tree/osseu17/slides/common/sampleapp.md)] --- ## Let's draw hasty conclusions - The bottleneck seems to be `rng` - *What if* we don't have enough entropy and can't generate enough random numbers? - We need to scale out the `rng` service on multiple machines! Note: this is a fiction! We have enough entropy. But we need a pretext to scale out. (In fact, the code of `rng` uses `/dev/urandom`, which never runs out of entropy...
...and is [just as good as `/dev/random`](http://www.slideshare.net/PacSecJP/filippo-plain-simple-reality-of-entropy).) .debug[[common/sampleapp.md](https://github.com/jpetazzo/container.training/tree/osseu17/slides/common/sampleapp.md)] --- ## Clean up - Before moving on, let's remove those containers .exercise[ - Tell Compose to remove everything: ```bash docker-compose down ``` ] .debug[[common/sampleapp.md](https://github.com/jpetazzo/container.training/tree/osseu17/slides/common/sampleapp.md)] --- name: toc-kubernetes-concepts class: title Kubernetes concepts .nav[[Back to table of contents](#toc-chapter-1)] .debug[(automatically generated title slide)] --- # Kubernetes concepts - Kubernetes is a container management system - It runs and manages containerized applications on a cluster -- - What does that really mean? .debug[[kube/concepts-k8s.md](https://github.com/jpetazzo/container.training/tree/osseu17/slides/kube/concepts-k8s.md)] --- ## Basic things we can ask Kubernetes to do -- - Start 5 containers using image `atseashop/api:v1.3` -- - Place an internal load balancer in front of these containers -- - Start 10 containers using image `atseashop/webfront:v1.3` -- - Place a public load balancer in front of these containers -- - It's Black Friday (or Christmas), traffic spikes, grow our cluster and add containers -- - New release! Replace my containers with the new image `atseashop/webfront:v1.4` -- - Keep processing requests during the upgrade; update my containers one at a time .debug[[kube/concepts-k8s.md](https://github.com/jpetazzo/container.training/tree/osseu17/slides/kube/concepts-k8s.md)] --- ## Other things that Kubernetes can do for us - Basic autoscaling - Blue/green deployment, canary deployment - Long running services, but also batch (one-off) jobs - Overcommit our cluster and *evict* low-priority jobs - Run services with *stateful* data (databases etc.) - Fine-grained access control defining *what* can be done by *whom* on *which* resources - Integrating third party services (*service catalog*) - Automating complex tasks (*operators*) .debug[[kube/concepts-k8s.md](https://github.com/jpetazzo/container.training/tree/osseu17/slides/kube/concepts-k8s.md)] --- ## Kubernetes architecture .debug[[kube/concepts-k8s.md](https://github.com/jpetazzo/container.training/tree/osseu17/slides/kube/concepts-k8s.md)] --- class: pic 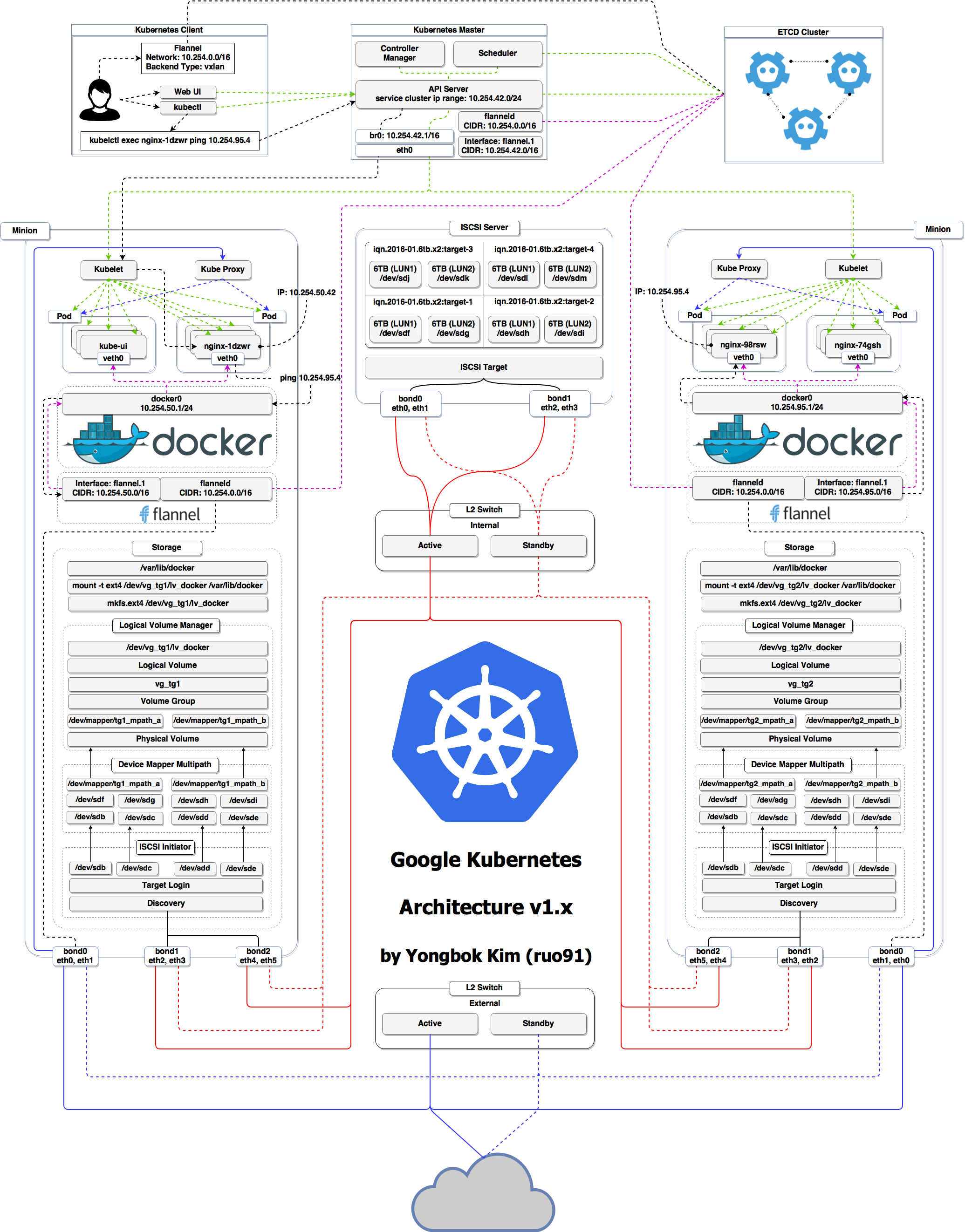 .debug[[kube/concepts-k8s.md](https://github.com/jpetazzo/container.training/tree/osseu17/slides/kube/concepts-k8s.md)] --- ## Kubernetes architecture - Ha ha ha ha - OK, I was trying to scare you, it's much simpler than that ❤️ .debug[[kube/concepts-k8s.md](https://github.com/jpetazzo/container.training/tree/osseu17/slides/kube/concepts-k8s.md)] --- class: pic 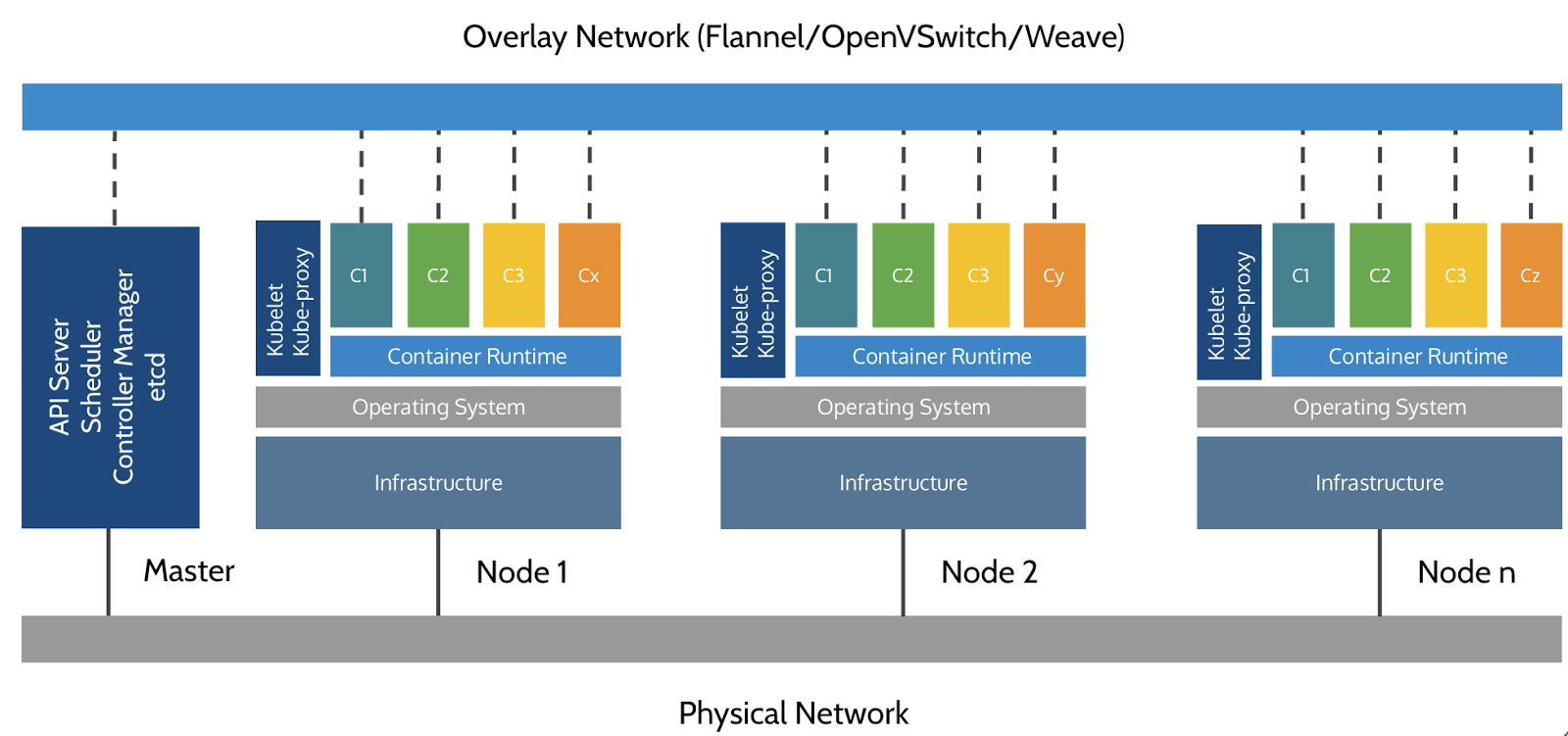 .debug[[kube/concepts-k8s.md](https://github.com/jpetazzo/container.training/tree/osseu17/slides/kube/concepts-k8s.md)] --- ## Credits - The first schema is a Kubernetes cluster with storage backed by multi-path iSCSI (Courtesy of [Yongbok Kim](https://www.yongbok.net/blog/)) - The second one is a simplified representation of a Kubernetes cluster (Courtesy of [Imesh Gunaratne](https://medium.com/containermind/a-reference-architecture-for-deploying-wso2-middleware-on-kubernetes-d4dee7601e8e)) .debug[[kube/concepts-k8s.md](https://github.com/jpetazzo/container.training/tree/osseu17/slides/kube/concepts-k8s.md)] --- ## Kubernetes architecture: the master - The Kubernetes logic (its "brains") is a collection of services: - the API server (our point of entry to everything!) - core services like the scheduler and controller manager - `etcd` (a highly available key/value store; the "database" of Kubernetes) - Together, these services form what is called the "master" - These services can run straight on a host, or in containers
(that's an implementation detail) - `etcd` can be run on separate machines (first schema) or co-located (second schema) - We need at least one master, but we can have more (for high availability) .debug[[kube/concepts-k8s.md](https://github.com/jpetazzo/container.training/tree/osseu17/slides/kube/concepts-k8s.md)] --- ## Kubernetes architecture: the nodes - The nodes executing our containers run another collection of services: - a container Engine (typically Docker) - kubelet (the "node agent") - kube-proxy (a necessary but not sufficient network component) - Nodes were formerly called "minions" - It is customary to *not* run apps on the node(s) running master components (Except when using small development clusters) .debug[[kube/concepts-k8s.md](https://github.com/jpetazzo/container.training/tree/osseu17/slides/kube/concepts-k8s.md)] --- ## Do we need to run Docker at all? No! -- - By default, Kubernetes uses the Docker Engine to run containers - We could also use `rkt` ("Rocket") from CoreOS - Or leverage other pluggable runtimes through the *Container Runtime Interface* (like CRI-O, or containerd) .debug[[kube/concepts-k8s.md](https://github.com/jpetazzo/container.training/tree/osseu17/slides/kube/concepts-k8s.md)] --- ## Do we need to run Docker at all? Yes! -- - In this workshop, we run our app on a single node first - We will need to build images and ship them around - We can do these things without Docker
(and get diagnosed with NIH¹ syndrome) - Docker is still the most stable container engine today
(but other options are maturing very quickly) .footnote[¹[Not Invented Here](https://en.wikipedia.org/wiki/Not_invented_here)] .debug[[kube/concepts-k8s.md](https://github.com/jpetazzo/container.training/tree/osseu17/slides/kube/concepts-k8s.md)] --- ## Do we need to run Docker at all? - On our development environments, CI pipelines ... : *Yes, almost certainly* - On our production servers: *Yes (today)* *Probably not (in the future)* .footnote[More information about CRI [on the Kubernetes blog](http://blog.kubernetes.io/2016/12/]container-runtime-interface-cri-in-kubernetes.html). .debug[[kube/concepts-k8s.md](https://github.com/jpetazzo/container.training/tree/osseu17/slides/kube/concepts-k8s.md)] --- ## Kubernetes resources - The Kubernetes API defines a lot of objects called *resources* - These resources are organized by type, or `Kind` (in the API) - A few common resource types are: - node (a machine — physical or virtual — in our cluster) - pod (group of containers running together on a node) - service (stable network endpoint to connect to one or multiple containers) - namespace (more-or-less isolated group of things) - secret (bundle of sensitive data to be passed to a container) And much more! (We can see the full list by running `kubectl get`) .debug[[kube/concepts-k8s.md](https://github.com/jpetazzo/container.training/tree/osseu17/slides/kube/concepts-k8s.md)] --- class: pic 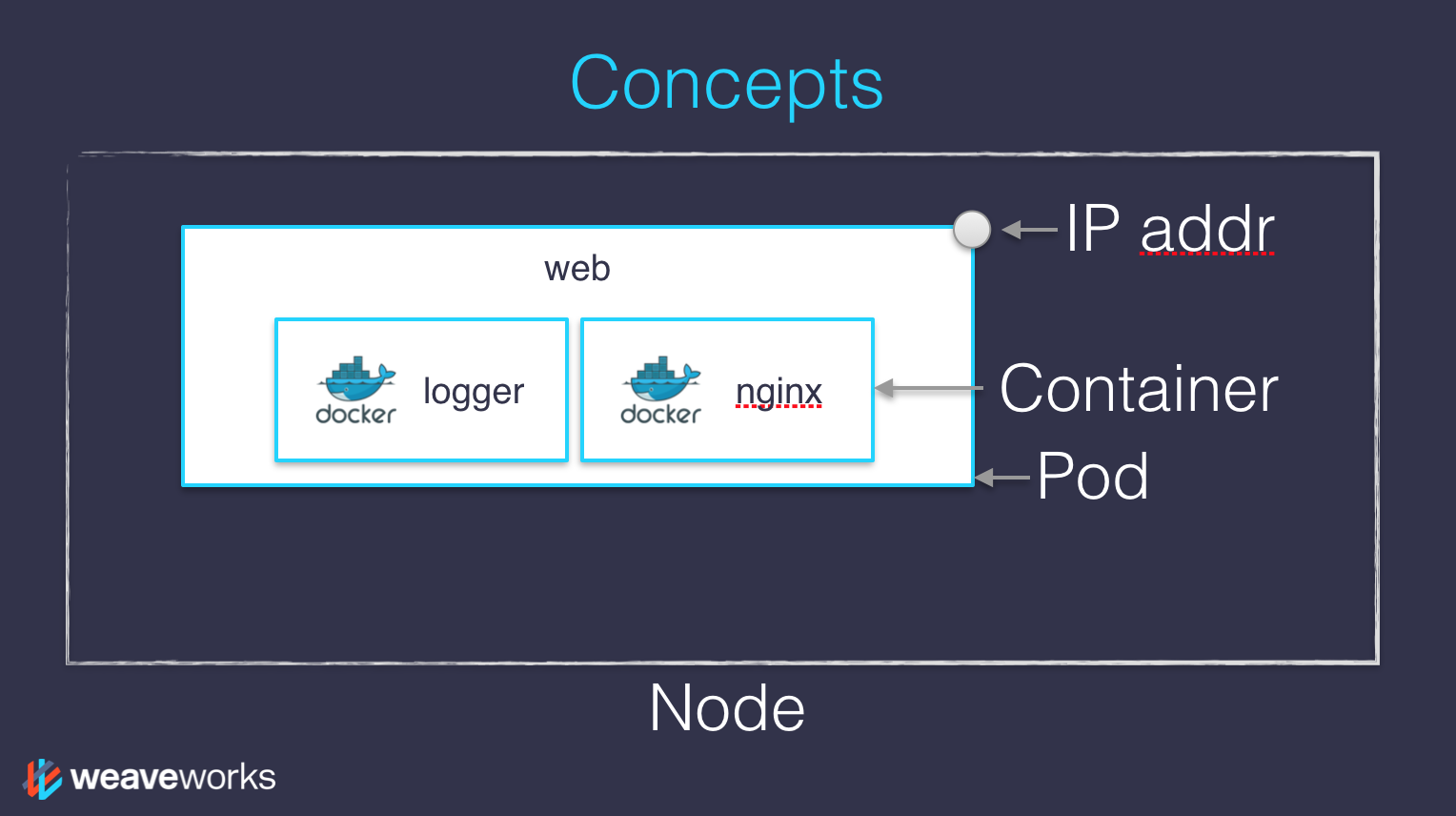 (Diagram courtesy of Weave Works, used with permission.) .debug[[kube/concepts-k8s.md](https://github.com/jpetazzo/container.training/tree/osseu17/slides/kube/concepts-k8s.md)] --- name: toc-declarative-vs-imperative class: title Declarative vs imperative .nav[[Back to table of contents](#toc-chapter-1)] .debug[(automatically generated title slide)] --- # Declarative vs imperative - Kubernetes puts a very strong emphasis on being *declarative* - Declarative: *I would like a cup of tea.* - Imperative: *Boil some water. Pour it in a teapot. Add tea leaves. Steep for a while. Serve in cup.* -- - Declarative seems simpler at first ... -- - ... As long as you know how to brew tea .debug[[kube/concepts-k8s.md](https://github.com/jpetazzo/container.training/tree/osseu17/slides/kube/concepts-k8s.md)] --- ## Declarative vs imperative - What declarative would really be: *I want a cup of tea, obtained by pouring an infusion¹ of tea leaves in a cup.* -- *¹An infusion is obtained by letting the object steep a few minutes in hot² water.* -- *²Hot liquid is obtained by pouring it in an appropriate container³ and setting it on a stove.* -- *³Ah, finally, containers! Something we know about. Let's get to work, shall we?* -- .footnote[Did you know there was an [ISO standard](https://en.wikipedia.org/wiki/ISO_3103) specifying how to brew tea?] .debug[[kube/concepts-k8s.md](https://github.com/jpetazzo/container.training/tree/osseu17/slides/kube/concepts-k8s.md)] --- ## Declarative vs imperative - Imperative systems: - simpler - if a task is interrupted, we have to restart from scratch - Declarative systems: - if a task is interrupted (or if we show up to the party half-way through), we can figure out what's missing and do only what's necessary - we need to be able to *observe* the system - ... and compute a "diff" between *what we have* and *what we want* .debug[[kube/concepts-k8s.md](https://github.com/jpetazzo/container.training/tree/osseu17/slides/kube/concepts-k8s.md)] --- ## Declarative vs imperative in Kubernetes - Virtually everything we create in Kubernetes is created from a *spec* - Watch for the `spec` fields in the YAML files later! - The *spec* describes *how we want the thing to be* - Kubernetes will *reconcile* the current state with the spec
(technically, this is done by a number of *controllers*) - When we want to change some resource, we update the *spec* - Kubernetes will then *converge* that resource .debug[[kube/concepts-k8s.md](https://github.com/jpetazzo/container.training/tree/osseu17/slides/kube/concepts-k8s.md)] --- name: toc-kubernetes-network-model class: title Kubernetes network model .nav[[Back to table of contents](#toc-chapter-1)] .debug[(automatically generated title slide)] --- # Kubernetes network model - TL,DR: *Our cluster (nodes and pods) is one big flat IP network.* -- - In detail: - all nodes must be able to reach each other, without NAT - all pods must be able to reach each other, without NAT - pods and nodes must be able to reach each other, without NAT - each pod is aware of its IP address (no NAT) - Kubernetes doesn't mandate any particular implementation .debug[[kube/kubenet.md](https://github.com/jpetazzo/container.training/tree/osseu17/slides/kube/kubenet.md)] --- ## Kubernetes network model: the good - Everything can reach everything - No address translation - No port translation - No new protocol - Pods cannot move from a node to another and keep their IP address - IP addresses don't have to be "portable" from a node to another (We can use e.g. a subnet per node and use a simple routed topology) - The specification is simple enough to allow many various implementations .debug[[kube/kubenet.md](https://github.com/jpetazzo/container.training/tree/osseu17/slides/kube/kubenet.md)] --- ## Kubernetes network model: the bad and the ugly - Everything can reach everything - if you want security, you need to add network policies - the network implementation that you use needs to support them - There are literally dozens of implementations out there (15 are listed in the Kubernetes documentation) - It *looks like* you have a level 3 network, but it's only level 4 (The spec requires UDP and TCP, but not port ranges or arbitrary IP packets) - `kube-proxy` is on the data path when connecting to a pod or container,
and it's not particularly fast (relies on userland proxying or iptables) .debug[[kube/kubenet.md](https://github.com/jpetazzo/container.training/tree/osseu17/slides/kube/kubenet.md)] --- ## Kubernetes network model: in practice - The nodes that we are using have been set up to use Weave - We don't endorse Weave in a particular way, it just Works For Us - Don't worry about the warning about `kube-proxy` performance - Unless you: - routinely saturate 10G network interfaces - count packet rates in millions per second - run high-traffic VOIP or gaming platforms - do weird things that involve millions of simultaneous connections
(in which case you're already familiar with kernel tuning) .debug[[kube/kubenet.md](https://github.com/jpetazzo/container.training/tree/osseu17/slides/kube/kubenet.md)] --- name: toc-first-contact-with-kubectl class: title First contact with `kubectl` .nav[[Back to table of contents](#toc-chapter-1)] .debug[(automatically generated title slide)] --- # First contact with `kubectl` - `kubectl` is (almost) the only tool we'll need to talk to Kubernetes - It is a rich CLI tool around the Kubernetes API (Everything you can do with `kubectl`, you can do directly with the API) - On our machines, there is a `~/.kube/config` file with: - the Kubernetes API address - the path to our TLS certificates used to authenticate - You can also use the `--kubeconfig` flag to pass a config file - Or directly `--server`, `--user`, etc. - `kubectl` can be pronounced "Cube C T L", "Cube cuttle", "Cube cuddle"... .debug[[kube/kubectlget.md](https://github.com/jpetazzo/container.training/tree/osseu17/slides/kube/kubectlget.md)] --- ## `kubectl get` - Let's look at our `Node` resources with `kubectl get`! .exercise[ - Look at the composition of our cluster: ```bash kubectl get node ``` - These commands are equivalent: ```bash kubectl get no kubectl get node kubectl get nodes ``` ] .debug[[kube/kubectlget.md](https://github.com/jpetazzo/container.training/tree/osseu17/slides/kube/kubectlget.md)] --- ## From human-readable to machine-readable output - `kubectl get` can output JSON, YAML, or be directly formatted .exercise[ - Give us more info about them nodes: ```bash kubectl get nodes -o wide ``` - Let's have some YAML: ```bash kubectl get no -o yaml ``` See that `kind: List` at the end? It's the type of our result! ] .debug[[kube/kubectlget.md](https://github.com/jpetazzo/container.training/tree/osseu17/slides/kube/kubectlget.md)] --- ## (Ab)using `kubectl` and `jq` - It's super easy to build custom reports .exercise[ - Show the capacity of all our nodes as a stream of JSON objects: ```bash kubectl get nodes -o json | jq ".items[] | {name:.metadata.name} + .status.capacity" ``` ] .debug[[kube/kubectlget.md](https://github.com/jpetazzo/container.training/tree/osseu17/slides/kube/kubectlget.md)] --- ## What's available? - `kubectl` has pretty good introspection facilities - We can list all available resource types by running `kubectl get` - We can view details about a resource with: ```bash kubectl describe type/name kubectl describe type name ``` - We can view the definition for a resource type with: ```bash kubectl explain type ``` Each time, `type` can be singular, plural, or abbreviated type name. .debug[[kube/kubectlget.md](https://github.com/jpetazzo/container.training/tree/osseu17/slides/kube/kubectlget.md)] --- ## Services - A *service* is a stable endpoint to connect to "something" (In the initial proposal, they were called "portals") .exercise[ - List the services on our cluster with one of these commands: ```bash kubectl get services kubectl get svc ``` ] -- There is already one service on our cluster: the Kubernetes API itself. .debug[[kube/kubectlget.md](https://github.com/jpetazzo/container.training/tree/osseu17/slides/kube/kubectlget.md)] --- ## ClusterIP services - A `ClusterIP` service is internal, available from the cluster only - This is useful for introspection from within containers .exercise[ - Try to connect to the API: ```bash curl -k https://`10.96.0.1` ``` - `-k` is used to skip certificate verification - Make sure to replace 10.96.0.1 with the CLUSTER-IP shown earlier ] -- The error that we see is expected: the Kubernetes API requires authentication. .debug[[kube/kubectlget.md](https://github.com/jpetazzo/container.training/tree/osseu17/slides/kube/kubectlget.md)] --- ## Listing running containers - Containers are manipulated through *pods* - A pod is a group of containers: - running together (on the same node) - sharing resources (RAM, CPU; but also network, volumes) .exercise[ - List pods on our cluster: ```bash kubectl get pods ``` ] -- *These are not the pods you're looking for.* But where are they?!? .debug[[kube/kubectlget.md](https://github.com/jpetazzo/container.training/tree/osseu17/slides/kube/kubectlget.md)] --- ## Namespaces - Namespaces allow to segregate resources .exercise[ - List the namespaces on our cluster with one of these commands: ```bash kubectl get namespaces kubectl get namespace kubectl get ns ``` ] -- *You know what ... This `kube-system` thing looks suspicious.* .debug[[kube/kubectlget.md](https://github.com/jpetazzo/container.training/tree/osseu17/slides/kube/kubectlget.md)] --- ## Accessing namespaces - By default, `kubectl` uses the `default` namespace - We can switch to a different namespace with the `-n` option .exercise[ - List the pods in the `kube-system` namespace: ```bash kubectl -n kube-system get pods ``` ] -- *Ding ding ding ding ding!* .debug[[kube/kubectlget.md](https://github.com/jpetazzo/container.training/tree/osseu17/slides/kube/kubectlget.md)] --- ## What are all these pods? - `etcd` is our etcd server - `kube-apiserver` is the API server - `kube-controller-manager` and `kube-scheduler` are other master components - `kube-dns` is an additional component (not mandatory but super useful, so it's there) - `kube-proxy` is the (per-node) component managing port mappings and such - `weave` is the (per-node) component managing the network overlay - the `READY` column indicates the number of containers in each pod - the pods with a name ending with `-node1` are the master components
(they have been specifically "pinned" to the master node) .debug[[kube/kubectlget.md](https://github.com/jpetazzo/container.training/tree/osseu17/slides/kube/kubectlget.md)] --- name: toc-setting-up-kubernetes class: title Setting up Kubernetes .nav[[Back to table of contents](#toc-chapter-1)] .debug[(automatically generated title slide)] --- # Setting up Kubernetes - How did we set up these Kubernetes clusters that we're using? -- - We used `kubeadm` on "fresh" EC2 instances with Ubuntu 16.04 LTS 1. Install Docker 2. Install Kubernetes packages 3. Run `kubeadm init` on the master node 4. Set up Weave (the overlay network)
(that step is just one `kubectl apply` command; discussed later) 5. Run `kubeadm join` on the other nodes (with the token produced by `kubeadm init`) 6. Copy the configuration file generated by `kubeadm init` .debug[[kube/setup-k8s.md](https://github.com/jpetazzo/container.training/tree/osseu17/slides/kube/setup-k8s.md)] --- ## `kubeadm` drawbacks - Doesn't set up Docker or any other container engine - Doesn't set up the overlay network - Scripting is complex
(because extracting the token requires advanced `kubectl` commands) - Doesn't set up multi-master (no high availability) -- - It's still twice as many steps as setting up a Swarm cluster 😕 .debug[[kube/setup-k8s.md](https://github.com/jpetazzo/container.training/tree/osseu17/slides/kube/setup-k8s.md)] --- ## Other deployment options - If you are on Google Cloud: [GKE](https://cloud.google.com/container-engine/) Empirically the best Kubernetes deployment out there - If you are on AWS: [kops](https://github.com/kubernetes/kops) ... But with AWS re:invent just around the corner, expect some changes - On a local machine: [minikube](https://kubernetes.io/docs/getting-started-guides/minikube/), [kubespawn](https://github.com/kinvolk/kube-spawn), [Docker4Mac (coming soon)](https://beta.docker.com/) - If you want something customizable: [kubicorn](https://github.com/kris-nova/kubicorn) Probably the closest to a multi-cloud/hybrid solution so far, but in development - Also, many commercial options! .debug[[kube/setup-k8s.md](https://github.com/jpetazzo/container.training/tree/osseu17/slides/kube/setup-k8s.md)] --- name: toc-running-our-first-containers-on-kubernetes class: title Running our first containers on Kubernetes .nav[[Back to table of contents](#toc-chapter-1)] .debug[(automatically generated title slide)] --- # Running our first containers on Kubernetes - First things first: we cannot run a container -- - We are going to run a pod, and in that pod there will be a single container -- - In that container in the pod, we are going to run a simple `ping` command - Then we are going to start additional copies of the pod .debug[[kube/kubectlrun.md](https://github.com/jpetazzo/container.training/tree/osseu17/slides/kube/kubectlrun.md)] --- ## Starting a simple pod with `kubectl run` - We need to specify at least a *name* and the image we want to use .exercise[ - Let's ping `goo.gl`: ```bash kubectl run pingpong --image alpine ping goo.gl ``` ] -- OK, what just happened? .debug[[kube/kubectlrun.md](https://github.com/jpetazzo/container.training/tree/osseu17/slides/kube/kubectlrun.md)] --- ## Behind the scenes of `kubectl run` - Let's look at the resources that were created by `kubectl run` .exercise[ - List most resource types: ```bash kubectl get all ``` ] -- We should see the following things: - `deploy/pingpong` (the *deployment* that we just created) - `rs/pingpong-xxxx` (a *replica set* created by the deployment) - `po/pingpong-yyyy` (a *pod* created by the replica set) .debug[[kube/kubectlrun.md](https://github.com/jpetazzo/container.training/tree/osseu17/slides/kube/kubectlrun.md)] --- ## Deployments, replica sets, and replication controllers - A *deployment* is a high-level construct - allows scaling, rolling updates, rollbacks - multiple deployments can be used together to implement a [canary deployment](https://kubernetes.io/docs/concepts/cluster-administration/manage-deployment/#canary-deployments) - delegates pods management to *replica sets* - A *replica set* is a low-level construct - makes sure that a given number of identical pods are running - allows scaling - rarely used directly - A *replication controller* is the (deprecated) predecessor of a replica set .debug[[kube/kubectlrun.md](https://github.com/jpetazzo/container.training/tree/osseu17/slides/kube/kubectlrun.md)] --- ## Our `pingpong` deployment - `kubectl run` created a *deployment*, `deploy/pingpong` - That deployment created a *replica set*, `rs/pingpong-xxxx` - That replica set created a *pod*, `po/pingpong-yyyy` - We'll see later how these folks play together for: - scaling - high availability - rolling updates .debug[[kube/kubectlrun.md](https://github.com/jpetazzo/container.training/tree/osseu17/slides/kube/kubectlrun.md)] --- ## Viewing container output - Let's use the `kubectl logs` command - We will pass either a *pod name*, or a *type/name* (E.g. if we specify a deployment or replica set, it will get the first pod in it) - Unless specified otherwise, it will only show logs of the first container in the pod (Good thing there's only one in ours!) .exercise[ - View the result of our `ping` command: ```bash kubectl logs deploy/pingpong ``` ] .debug[[kube/kubectlrun.md](https://github.com/jpetazzo/container.training/tree/osseu17/slides/kube/kubectlrun.md)] --- ## Streaming logs in real time - Just like `docker logs`, `kubectl logs` supports convenient options: - `-f`/`--follow` to stream logs in real time (à la `tail -f`) - `--tail` to indicate how many lines you want to see (from the end) - `--since` to get logs only after a given timestamp .exercise[ - View the latest logs of our `ping` command: ```bash kubectl logs deploy/pingpong --tail 1 --follow ``` ] .debug[[kube/kubectlrun.md](https://github.com/jpetazzo/container.training/tree/osseu17/slides/kube/kubectlrun.md)] --- ## Scaling our application - We can create additional copies of our container (I mean, our pod) with `kubectl scale` .exercise[ - Scale our `pingpong` deployment: ```bash kubectl scale deploy/pingpong --replicas 8 ``` ] Note: what if we tried to scale `rs/pingpong-xxxx`? We could! But the *deployment* would notice it right away, and scale back to the initial level. .debug[[kube/kubectlrun.md](https://github.com/jpetazzo/container.training/tree/osseu17/slides/kube/kubectlrun.md)] --- ## Resilience - The *deployment* `pingpong` watches its *replica set* - The *replica set* ensures that the right number of *pods* are running - What happens if pods disappear? .exercise[ - In a separate window, list pods, and keep watching them: ```bash kubectl get pods -w ``` - Destroy a pod: ```bash kubectl delete pod pingpong-yyyy ``` ] .debug[[kube/kubectlrun.md](https://github.com/jpetazzo/container.training/tree/osseu17/slides/kube/kubectlrun.md)] --- ## What if we wanted something different? - What if we wanted to start a "one-shot" container that *doesn't* get restarted? - We could use `kubectl run --restart=OnFailure` or `kubectl run --restart=Never` - These commands would create *jobs* or *pods* instead of *deployments* - Under the hood, `kubectl run` invokes "generators" to create resource descriptions - We could also write these resource descriptions ourselves (typically in YAML),
and create them on the cluster with `kubectl apply -f` (discussed later) - With `kubectl run --schedule=...`, we can also create *cronjobs* .debug[[kube/kubectlrun.md](https://github.com/jpetazzo/container.training/tree/osseu17/slides/kube/kubectlrun.md)] --- ## Viewing logs of multiple pods - When we specify a deployment name, only one single pod's logs are shown - We can view the logs of multiple pods by specifying a *selector* - A selector is a logic expression using *labels* - Conveniently, when you `kubectl run somename`, the associated objects have a `run=somename` label .exercise[ - View the last line of log from all pods with the `run=pingpong` label: ```bash kubectl logs -l run=pingpong --tail 1 ``` ] Unfortunately, `--follow` cannot (yet) be used to stream the logs from multiple containers. .debug[[kube/kubectlrun.md](https://github.com/jpetazzo/container.training/tree/osseu17/slides/kube/kubectlrun.md)] --- class: title .small[ Meanwhile, at the Google NOC ... .small[ Why the hell
are we getting 1000 packets per second
of ICMP ECHO traffic from EC2 ?!? ] ] .debug[[kube/kubectlrun.md](https://github.com/jpetazzo/container.training/tree/osseu17/slides/kube/kubectlrun.md)] --- name: toc-exposing-containers class: title Exposing containers .nav[[Back to table of contents](#toc-chapter-1)] .debug[(automatically generated title slide)] --- # Exposing containers - `kubectl expose` creates a *service* for existing pods - A *service* is a stable address for a pod (or a bunch of pods) - If we want to connect to our pod(s), we need to create a *service* - Once a service is created, `kube-dns` will allow us to resolve it by name (i.e. after creating service `hello`, the name `hello` will resolve to something) - There are different types of services, detailed on the following slides: `ClusterIP`, `NodePort`, `LoadBalancer`, `ExternalName` .debug[[kube/kubectlexpose.md](https://github.com/jpetazzo/container.training/tree/osseu17/slides/kube/kubectlexpose.md)] --- ## Basic service types - `ClusterIP` (default type) - a virtual IP address is allocated for the service (in an internal, private range) - this IP address is reachable only from within the cluster (nodes and pods) - our code can connect to the service using the original port number - `NodePort` - a port is allocated for the service (by default, in the 30000-32768 range) - that port is made available *on all our nodes* and anybody can connect to it - our code must be changed to connect to that new port number These service types are always available. Under the hood: `kube-proxy` is using a userland proxy and a bunch of `iptables` rules. .debug[[kube/kubectlexpose.md](https://github.com/jpetazzo/container.training/tree/osseu17/slides/kube/kubectlexpose.md)] --- ## More service types - `LoadBalancer` - an external load balancer is allocated for the service - the load balancer is configured accordingly
(e.g.: a `NodePort` service is created, and the load balancer sends traffic to that port) - `ExternalName` - the DNS entry managed by `kube-dns` will just be a `CNAME` to a provided record - no port, no IP address, no nothing else is allocated The `LoadBalancer` type is currently only available on AWS, Azure, and GCE. .debug[[kube/kubectlexpose.md](https://github.com/jpetazzo/container.training/tree/osseu17/slides/kube/kubectlexpose.md)] --- ## Running containers with open ports - Since `ping` doesn't have anything to connect to, we'll have to run something else .exercise[ - Start a bunch of ElasticSearch containers: ```bash kubectl run elastic --image=elasticsearch:2 --replicas=7 ``` - Watch them being started: ```bash kubectl get pods -w ``` ] The `-w` option "watches" events happening on the specified resources. Note: please DO NOT call the service `search`. It would collide with the TLD. .debug[[kube/kubectlexpose.md](https://github.com/jpetazzo/container.training/tree/osseu17/slides/kube/kubectlexpose.md)] --- ## Exposing our deployment - We'll create a default `ClusterIP` service .exercise[ - Expose the ElasticSearch HTTP API port: ```bash kubectl expose deploy/elastic --port 9200 ``` - Look up which IP address was allocated: ```bash kubectl get svc ``` ] .debug[[kube/kubectlexpose.md](https://github.com/jpetazzo/container.training/tree/osseu17/slides/kube/kubectlexpose.md)] --- ## Services are layer 4 constructs - You can assign IP addresses to services, but they are still *layer 4* (i.e. a service is not an IP address; it's an IP address + protocol + port) - This is caused by the current implementation of `kube-proxy` (it relies on mechanisms that don't support layer 3) - As a result: you *have to* indicate the port number for your service - Running services with arbitrary port (or port ranges) requires hacks (e.g. host networking mode) .debug[[kube/kubectlexpose.md](https://github.com/jpetazzo/container.training/tree/osseu17/slides/kube/kubectlexpose.md)] --- ## Testing our service - We will now send a few HTTP requests to our ElasticSearch pods .exercise[ - Let's obtain the IP address that was allocated for our service, *programatically:* ```bash IP=$(kubectl get svc elastic -o go-template --template '{{ .spec.clusterIP }}') ``` - Send a few requests: ```bash curl http://$IP:9200/ ``` ] -- Our requests are load balanced across multiple pods. .debug[[kube/kubectlexpose.md](https://github.com/jpetazzo/container.training/tree/osseu17/slides/kube/kubectlexpose.md)] --- class: title Our app on Kube .debug[[kube/ourapponkube.md](https://github.com/jpetazzo/container.training/tree/osseu17/slides/kube/ourapponkube.md)] --- ## What's on the menu? In this part, we will: - **build** images for our app, - **ship** these images with a registry, - **run** deployments using these images, - expose these deployments so they can communicate with each other, - expose the web UI so we can access it from outside. .debug[[kube/ourapponkube.md](https://github.com/jpetazzo/container.training/tree/osseu17/slides/kube/ourapponkube.md)] --- ## The plan - Build on our control node (`node1`) - Tag images so that they are named `$REGISTRY/servicename` - Upload them to a registry - Create deployments using the images - Expose (with a ClusterIP) the services that need to communicate - Expose (with a NodePort) the WebUI .debug[[kube/ourapponkube.md](https://github.com/jpetazzo/container.training/tree/osseu17/slides/kube/ourapponkube.md)] --- ## Which registry do we want to use? - We could use the Docker Hub - Or a service offered by our cloud provider (GCR, ECR...) - Or we could just self-host that registry *We'll self-host the registry because it's the most generic solution for this workshop.* .debug[[kube/ourapponkube.md](https://github.com/jpetazzo/container.training/tree/osseu17/slides/kube/ourapponkube.md)] --- ## Using the open source registry - We need to run a `registry:2` container
(make sure you specify tag `:2` to run the new version!) - It will store images and layers to the local filesystem
(but you can add a config file to use S3, Swift, etc.) - Docker *requires* TLS when communicating with the registry - unless for registries on `127.0.0.0/8` (i.e. `localhost`) - or with the Engine flag `--insecure-registry` - Our strategy: publish the registry container on a NodePort,
so that it's available through `127.0.0.1:xxxxx` on each node .debug[[kube/ourapponkube.md](https://github.com/jpetazzo/container.training/tree/osseu17/slides/kube/ourapponkube.md)] --- name: toc-deploying-a-self-hosted-registry class: title Deploying a self-hosted registry .nav[[Back to table of contents](#toc-chapter-1)] .debug[(automatically generated title slide)] --- # Deploying a self-hosted registry - We will deploy a registry container, and expose it with a NodePort .exercise[ - Create the registry service: ```bash kubectl run registry --image=registry:2 ``` - Expose it on a NodePort: ```bash kubectl expose deploy/registry --port=5000 --type=NodePort ``` ] .debug[[kube/ourapponkube.md](https://github.com/jpetazzo/container.training/tree/osseu17/slides/kube/ourapponkube.md)] --- ## Connecting to our registry - We need to find out which port has been allocated .exercise[ - View the service details: ```bash kubectl describe svc/registry ``` - Get the port number programmatically: ```bash NODEPORT=$(kubectl get svc/registry -o json | jq .spec.ports[0].nodePort) REGISTRY=127.0.0.1:$NODEPORT ``` ] .debug[[kube/ourapponkube.md](https://github.com/jpetazzo/container.training/tree/osseu17/slides/kube/ourapponkube.md)] --- ## Testing our registry - A convenient Docker registry API route to remember is `/v2/_catalog` .exercise[ - View the repositories currently held in our registry: ```bash curl $REGISTRY/v2/_catalog ``` ] -- We should see: ```json {"repositories":[]} ``` .debug[[kube/ourapponkube.md](https://github.com/jpetazzo/container.training/tree/osseu17/slides/kube/ourapponkube.md)] --- ## Testing our local registry - We can retag a small image, and push it to the registry .exercise[ - Make sure we have the busybox image, and retag it: ```bash docker pull busybox docker tag busybox $REGISTRY/busybox ``` - Push it: ```bash docker push $REGISTRY/busybox ``` ] .debug[[kube/ourapponkube.md](https://github.com/jpetazzo/container.training/tree/osseu17/slides/kube/ourapponkube.md)] --- ## Checking again what's on our local registry - Let's use the same endpoint as before .exercise[ - Ensure that our busybox image is now in the local registry: ```bash curl $REGISTRY/v2/_catalog ``` ] The curl command should now output: ```json {"repositories":["busybox"]} ``` .debug[[kube/ourapponkube.md](https://github.com/jpetazzo/container.training/tree/osseu17/slides/kube/ourapponkube.md)] --- ## Building and pushing our images - We are going to use a convenient feature of Docker Compose .exercise[ - Go to the `stacks` directory: ```bash cd ~/orchestration-workshop/stacks ``` - Build and push the images: ```bash export REGISTRY docker-compose -f dockercoins.yml build docker-compose -f dockercoins.yml push ``` ] Let's have a look at the `dockercoins.yml` file while this is building and pushing. .debug[[kube/ourapponkube.md](https://github.com/jpetazzo/container.training/tree/osseu17/slides/kube/ourapponkube.md)] --- ```yaml version: "3" services: rng: build: dockercoins/rng image: ${REGISTRY-127.0.0.1:5000}/rng:${TAG-latest} deploy: mode: global ... redis: image: redis ... worker: build: dockercoins/worker image: ${REGISTRY-127.0.0.1:5000}/worker:${TAG-latest} ... deploy: replicas: 10 ``` .warning[Just in case you were wondering ... Docker "services" are not Kubernetes "services".] .debug[[kube/ourapponkube.md](https://github.com/jpetazzo/container.training/tree/osseu17/slides/kube/ourapponkube.md)] --- ## Deploying all the things - We can now deploy our code (as well as a redis instance) .exercise[ - Deploy `redis`: ```bash kubectl run redis --image=redis ``` - Deploy everything else: ```bash for SERVICE in hasher rng webui worker; do kubectl run $SERVICE --image=$REGISTRY/$SERVICE done ``` ] .debug[[kube/ourapponkube.md](https://github.com/jpetazzo/container.training/tree/osseu17/slides/kube/ourapponkube.md)] --- ## Is this working? - After waiting for the deployment to complete, let's look at the logs! (Hint: use `kubectl get deploy -w` to watch deployment events) .exercise[ - Look at some logs: ```bash kubectl logs deploy/rng kubectl logs deploy/worker ``` ] -- 🤔 `rng` is fine ... But not `worker`. -- 💡 Oh right! We forgot to `expose`. .debug[[kube/ourapponkube.md](https://github.com/jpetazzo/container.training/tree/osseu17/slides/kube/ourapponkube.md)] --- name: toc-exposing-services-internally- class: title Exposing services internally .nav[[Back to table of contents](#toc-chapter-1)] .debug[(automatically generated title slide)] --- # Exposing services internally - Three deployments need to be reachable by others: `hasher`, `redis`, `rng` - `worker` doesn't need to be exposed - `webui` will be dealt with later .exercise[ - Expose each deployment, specifying the right port: ```bash kubectl expose deployment redis --port 6379 kubectl expose deployment rng --port 80 kubectl expose deployment hasher --port 80 ``` ] .debug[[kube/ourapponkube.md](https://github.com/jpetazzo/container.training/tree/osseu17/slides/kube/ourapponkube.md)] --- ## Is this working yet? - The `worker` has an infinite loop, that retries 10 seconds after an error .exercise[ - Stream the worker's logs: ```bash kubectl logs deploy/worker --follow ``` (Give it about 10 seconds to recover) ] -- We should now see the `worker`, well, working happily. .debug[[kube/ourapponkube.md](https://github.com/jpetazzo/container.training/tree/osseu17/slides/kube/ourapponkube.md)] --- name: toc-exposing-services-for-external-access class: title Exposing services for external access .nav[[Back to table of contents](#toc-chapter-1)] .debug[(automatically generated title slide)] --- # Exposing services for external access - Now we would like to access the Web UI - We will expose it with a `NodePort` (just like we did for the registry) .exercise[ - Create a `NodePort` service for the Web UI: ```bash kubectl expose deploy/webui --type=NodePort --port=80 ``` - Check the port that was allocated: ```bash kubectl get svc ``` ] .debug[[kube/ourapponkube.md](https://github.com/jpetazzo/container.training/tree/osseu17/slides/kube/ourapponkube.md)] --- ## Accessing the web UI - We can now connect to *any node*, on the allocated node port, to view the web UI .exercise[ - Open the web UI in your browser (http://node-ip-address:3xxxx/) ] -- *Alright, we're back to where we started, when we were running on a single node!* .debug[[kube/ourapponkube.md](https://github.com/jpetazzo/container.training/tree/osseu17/slides/kube/ourapponkube.md)] --- name: toc-the-kubernetes-dashboard class: title The Kubernetes dashboard .nav[[Back to table of contents](#toc-chapter-1)] .debug[(automatically generated title slide)] --- # The Kubernetes dashboard - Kubernetes resources can also be viewed with a web dashboard - We are going to deploy that dashboard with *three commands:* - one to actually *run* the dashboard - one to make the dashboard available from outside - one to bypass authentication for the dashboard -- .footnote[.warning[Yes, this will open our cluster to all kinds of shenanigans. Don't do this at home.]] .debug[[kube/dashboard.md](https://github.com/jpetazzo/container.training/tree/osseu17/slides/kube/dashboard.md)] --- ## Running the dashboard - We need to create a *deployment* and a *service* for the dashboard - But also a *secret*, a *service account*, a *role* and a *role binding* - All these things can be defined in a YAML file and created with `kubectl apply -f` .exercise[ - Create all the dashboard resources, with the following command: ```bash kubectl apply -f https://goo.gl/Qamqab ``` ] The goo.gl URL expands to:
.small[https://raw.githubusercontent.com/kubernetes/dashboard/master/src/deploy/recommended/kubernetes-dashboard.yaml] .debug[[kube/dashboard.md](https://github.com/jpetazzo/container.training/tree/osseu17/slides/kube/dashboard.md)] --- ## Making the dashboard reachable from outside - The dashboard is exposed through a `ClusterIP` service - We need a `NodePort` service instead .exercise[ - Edit the service: ```bash kubectl edit service kubernetes-dashboard ``` ] -- `NotFound`?!? Y U NO WORK?!? .debug[[kube/dashboard.md](https://github.com/jpetazzo/container.training/tree/osseu17/slides/kube/dashboard.md)] --- ## Editing the `kubernetes-dashboard` service - If we look at the YAML that we loaded just before, we'll get a hint -- - The dashboard was created in the `kube-system` namespace .exercise[ - Edit the service: ```bash kubectl -n kube-system edit service kubernetes-dashboard ``` - Change `ClusterIP` to `NodePort`, save, and exit - Check the port that was assigned with `kubectl -n kube-system get services` ] .debug[[kube/dashboard.md](https://github.com/jpetazzo/container.training/tree/osseu17/slides/kube/dashboard.md)] --- ## Connecting to the dashboard .exercise[ - Connect to https://oneofournodes:3xxxx/ (You will have to work around the TLS certificate validation warning) ] - We have three authentication options at this point: - token (associated with a role that has appropriate permissions) - kubeconfig (e.g. using the `~/.kube/config` file from `node1`) - "skip" (use the dashboard "service account") - Let's use "skip": we get a bunch of warnings and don't see much .debug[[kube/dashboard.md](https://github.com/jpetazzo/container.training/tree/osseu17/slides/kube/dashboard.md)] --- ## Granting more rights to the dashboard - The dashboard documentation [explains how to do](https://github.com/kubernetes/dashboard/wiki/Access-control#admin-privileges) - We just need to load another YAML file! .exercise[ - Grant admin privileges to the dashboard so we can see our resources: ```bash kubectl apply -f https://goo.gl/CHsLTA ``` - Reload the dashboard and enjoy! ] -- .warning[By the way, we just added a backdoor to our Kubernetes cluster!] .debug[[kube/dashboard.md](https://github.com/jpetazzo/container.training/tree/osseu17/slides/kube/dashboard.md)] --- name: toc-security-implications-of-kubectl-apply class: title Security implications of `kubectl apply` .nav[[Back to table of contents](#toc-chapter-1)] .debug[(automatically generated title slide)] --- # Security implications of `kubectl apply` - When we do `kubectl apply -f
`, we create arbitrary resources - Resources can be evil; imagine a `deployment` that ... -- - starts bitcoin miners on the whole cluster -- - hides in a non-default namespace -- - bind-mounts our nodes' filesystem -- - inserts SSH keys in the root account (on the node) -- - encrypts our data and ransoms it -- - ☠️☠️☠️ .debug[[kube/dashboard.md](https://github.com/jpetazzo/container.training/tree/osseu17/slides/kube/dashboard.md)] --- ## `kubectl apply` is the new `curl | sh` - `curl | sh` is convenient - It's safe if you use HTTPS URLs from trusted sources -- - `kubectl apply -f` is convenient - It's safe if you use HTTPS URLs from trusted sources -- - It introduces new failure modes - Example: the official setup instructions for most pod networks .debug[[kube/dashboard.md](https://github.com/jpetazzo/container.training/tree/osseu17/slides/kube/dashboard.md)] --- name: toc-scaling-a-deployment class: title Scaling a deployment .nav[[Back to table of contents](#toc-chapter-1)] .debug[(automatically generated title slide)] --- # Scaling a deployment - We will start with an easy one: the `worker` deployment .exercise[ - Open two new terminals to check what's going on with pods and deployments: ```bash kubectl get pods -w kubectl get deployments -w ``` - Now, create more `worker` replicas: ```bash kubectl scale deploy/worker --replicas=10 ``` ] After a few seconds, the graph in the web UI should show up.
(And peak at 10 hashes/second, just like when we were running on a single one.) .debug[[kube/kubectlscale.md](https://github.com/jpetazzo/container.training/tree/osseu17/slides/kube/kubectlscale.md)] --- name: toc-daemon-sets class: title Daemon sets .nav[[Back to table of contents](#toc-chapter-1)] .debug[(automatically generated title slide)] --- # Daemon sets - Remember: we did all that cluster orchestration business for `rng` - We want one (and exactly one) instance of `rng` per node - If we just scale `deploy/rng` to 4, nothing guarantees that they spread - Instead of a `deployment`, we will use a `daemonset` - Daemon sets are great for cluster-wide, per-node processes: - `kube-proxy` - `weave` (our overlay network) - monitoring agents - hardware management tools (e.g. SCSI/FC HBA agents) - etc. - They can also be restricted to run [only on some nodes](https://kubernetes.io/docs/concepts/workloads/controllers/daemonset/#running-pods-on-only-some-nodes) .debug[[kube/daemonset.md](https://github.com/jpetazzo/container.training/tree/osseu17/slides/kube/daemonset.md)] --- ## Creating a daemon set - Unfortunately, as of Kubernetes 1.8, the CLI cannot create daemon sets -- - More precisely: it doesn't have a subcommand to create a daemon set -- - But any kind of resource can always be created by providing a YAML description: ```bash kubectl apply -f foo.yaml ``` -- - How do we create the YAML file for our daemon set? -- - option 1: read the docs -- - option 2: `vi` our way out of it .debug[[kube/daemonset.md](https://github.com/jpetazzo/container.training/tree/osseu17/slides/kube/daemonset.md)] --- ## Creating the YAML file for our daemon set - Let's start with the YAML file for the current `rng` resource .exercise[ - Dump the `rng` resource in YAML: ```bash kubectl get deploy/rng -o yaml --export >rng.yml ``` - Edit `rng.yml` ] Note: `--export` will remove "cluster-specific" information, i.e.: - namespace (so that the resource is not tied to a specific namespace) - status and creation timestamp (useless when creating a new resource) - resourceVersion and uid (these would cause... *interesting* problems) .debug[[kube/daemonset.md](https://github.com/jpetazzo/container.training/tree/osseu17/slides/kube/daemonset.md)] --- ## "Casting" a resource to another - What if we just changed the `kind` field? (It can't be that easy, right?) .exercise[ - Change `kind: Deployment` to `kind: DaemonSet` - Save, quit - Try to create our new resource: ```bash kubectl apply -f rng.yml ``` ] -- We all knew this couldn't be that easy, right! .debug[[kube/daemonset.md](https://github.com/jpetazzo/container.training/tree/osseu17/slides/kube/daemonset.md)] --- ## Understanding the problem - The core of the error is: ``` error validating data: [ValidationError(DaemonSet.spec): unknown field "replicas" in io.k8s.api.extensions.v1beta1.DaemonSetSpec, ... ``` -- - *Obviously,* it doesn't make sense to specify a number of replicas for a daemon set -- - Workaround: fix the YAML - remove the `replicas` field - remove the `strategy` field (which defines the rollout mechanism for a deployment) - remove the `status: {}` line at the end -- - Or, we could also ... .debug[[kube/daemonset.md](https://github.com/jpetazzo/container.training/tree/osseu17/slides/kube/daemonset.md)] --- ## Use the `--force`, Luke - We could also tell Kubernetes to ignore these errors and try anyway - The `--force` flag actual name is `--validate=false` .exercise[ - Try to load our YAML file and ignore errors: ```bash kubectl apply -f rng.yml --validate=false ``` ] -- 🎩✨🐇 -- Wait ... Now, can it be *that* easy? .debug[[kube/daemonset.md](https://github.com/jpetazzo/container.training/tree/osseu17/slides/kube/daemonset.md)] --- ## Checking what we've done - Did we transform our `deployment` into a `daemonset`? .exercise[ - Look at the resources that we have now: ```bash kubectl get all ``` ] -- We have both `deploy/rng` and `ds/rng` now! -- And one too many pods... .debug[[kube/daemonset.md](https://github.com/jpetazzo/container.training/tree/osseu17/slides/kube/daemonset.md)] --- ## Explanation - You can have different resource types with the same name (i.e. a *deployment* and a *daemonset* both named `rng`) - We still have the old `rng` *deployment* - But now we have the new `rng` *daemonset* as well - If we look at the pods, we have: - *one pod* for the deployment - *one pod per node* for the daemonset .debug[[kube/daemonset.md](https://github.com/jpetazzo/container.training/tree/osseu17/slides/kube/daemonset.md)] --- ## What are all these pods doing? - Let's check the logs of all these `rng` pods - All these pods have a `run=rng` label: - the first pod, because that's what `kubectl run` does - the other ones (in the daemon set), because we *copied the spec from the first one* - Therefore, we can query everybody's logs using that `run=rng` selector .exercise[ - Check the logs of all the pods having a label `run=rng`: ```bash kubectl logs -l run=rng --tail 1 ``` ] -- It appears that *all the pods* are serving requests at the moment. .debug[[kube/daemonset.md](https://github.com/jpetazzo/container.training/tree/osseu17/slides/kube/daemonset.md)] --- ## The magic of selectors - The `rng` *service* is load balancing requests to a set of pods - This set of pods is defined as "pods having the label `run=rng`" .exercise[ - Check the *selector* in the `rng` service definition: ```bash kubectl describe service rng ``` ] When we created additional pods with this label, they were automatically detected by `svc/rng` and added as *endpoints* to the associated load balancer. .debug[[kube/daemonset.md](https://github.com/jpetazzo/container.training/tree/osseu17/slides/kube/daemonset.md)] --- ## Removing the first pod from the load balancer - What would happen if we removed that pod, with `kubectl delete pod ...`? -- The `replicaset` would re-create it immediately. -- - What would happen if we removed the `run=rng` label from that pod? -- The `replicaset` would re-create it immediately. -- ... Because what matters to the `replicaset` is the number of pods *matching that selector.* -- - But but but ... Don't we have more than one pod with `run=rng` now? -- The answer lies in the exact selector used by the `replicaset` ... .debug[[kube/daemonset.md](https://github.com/jpetazzo/container.training/tree/osseu17/slides/kube/daemonset.md)] --- ## Deep dive into selectors - Let's look at the selectors for the `rng` *deployment* and the associated *replica set* .exercise[ - Show detailed information about the `rng` deployment: ```bash kubectl describe deploy rng ``` - Show detailed information about the `rng` replica:
(The second command doesn't require you to get the exact name of the replica set) ```bash kubectl describe rs rng-yyyy kubectl describe rs -l run=rng ``` ] -- The replica set selector also has a `pod-template-hash`, unlike the pods in our daemon set. .debug[[kube/daemonset.md](https://github.com/jpetazzo/container.training/tree/osseu17/slides/kube/daemonset.md)] --- name: toc-updating-a-service-through-labels-and-selectors class: title Updating a service through labels and selectors .nav[[Back to table of contents](#toc-chapter-1)] .debug[(automatically generated title slide)] --- # Updating a service through labels and selectors - What if we want to drop the `rng` deployment from the load balancer? - Option 1: - destroy it - Option 2: - add an extra *label* to the daemon set - update the service *selector* to refer to that *label* -- Of course, option 2 offers more learning opportunities. Right? .debug[[kube/daemonset.md](https://github.com/jpetazzo/container.training/tree/osseu17/slides/kube/daemonset.md)] --- ## Add an extra label to the daemon set - We will update the daemon set "spec" - Option 1: - edit the `rng.yml` file that we used earlier - load the new definition with `kubectl apply` - Option 2: - use `kubectl edit` -- *If you feel like you got this💕🌈, feel free to try directly.* *We've included a few hints on the next slides for your convenience!* .debug[[kube/daemonset.md](https://github.com/jpetazzo/container.training/tree/osseu17/slides/kube/daemonset.md)] --- ## We've put resources in your resources all the way down - Reminder: a daemon set is a resource that creates more resources! - There is a difference between: - the label(s) of a resource (in the `metadata` block in the beginning) - the selector of a resource (in the `spec` block) - the label(s) of the resource(s) created by the first resource (in the `template` block) - You need to update the selector and the template (metadata labels are not mandatory) - The template must match the selector (i.e. the resource will refuse to create resources that it will not select) .debug[[kube/daemonset.md](https://github.com/jpetazzo/container.training/tree/osseu17/slides/kube/daemonset.md)] --- ## Adding our label - Let's add a label `isactive: yes` - In YAML, `yes` should be quoted; i.e. `isactive: "yes"` .exercise[ - Update the daemon set to add `isactive: "yes"` to the selector and template label: ```bash kubectl edit daemonset rng ``` - Update the service to add `isactive: "yes"` to its selector: ```bash kubectl edit service rng ``` ] .debug[[kube/daemonset.md](https://github.com/jpetazzo/container.training/tree/osseu17/slides/kube/daemonset.md)] --- ## Checking what we've done .exercise[ - Check the logs of all `run=rng` pods to confirm that only 4 of them are now active: ```bash kubectl logs -l run=rng ``` ] The timestamps should give us a hint about how many pods are currently receiving traffic. .exercise[ - Look at the pods that we have right now: ```bash kubectl get pods ``` ] .debug[[kube/daemonset.md](https://github.com/jpetazzo/container.training/tree/osseu17/slides/kube/daemonset.md)] --- ## More labels, more selectors, more problems? - Bonus exercise 1: clean up the pods of the "old" daemon set - Bonus exercise 2: how could we have done to avoid creating new pods? .debug[[kube/daemonset.md](https://github.com/jpetazzo/container.training/tree/osseu17/slides/kube/daemonset.md)] --- name: toc-rolling-updates class: title Rolling updates .nav[[Back to table of contents](#toc-chapter-1)] .debug[(automatically generated title slide)] --- # Rolling updates - By default (without rolling updates), when a scaled resource is updated: - new pods are created - old pods are terminated - ... all at the same time - if something goes wrong, ¯\\\_(ツ)\_/¯ .debug[[kube/rollout.md](https://github.com/jpetazzo/container.training/tree/osseu17/slides/kube/rollout.md)] --- ## Rolling updates - With rolling updates, when a resource is updated, it happens progressively - Two parameters determine the pace of the rollout: `maxUnavailable` and `maxSurge` - They can be specified in absolute number of pods, or percentage of the `replicas` count - At any given time ... - there will always be at least `replicas`-`maxUnavailable` pods available - there will never be more than `replicas`+`maxSurge` pods in total - there will therefore be up to `maxUnavailable`+`maxSurge` pods being updated - We have the possibility to rollback to the previous version
(if the update fails or is unsatisfactory in any way) .debug[[kube/rollout.md](https://github.com/jpetazzo/container.training/tree/osseu17/slides/kube/rollout.md)] --- ## Rolling updates in practice - As of Kubernetes 1.8, we can do rolling updates with: `deployments`, `daemonsets`, `statefulsets` - Editing one of these resources will automatically result in a rolling update - Rolling updates can be monitored with the `kubectl rollout` subcommand .debug[[kube/rollout.md](https://github.com/jpetazzo/container.training/tree/osseu17/slides/kube/rollout.md)] --- ## Building a new version of the `worker` service .exercise[ - Go to the `stack` directory: ```bash cd ~/orchestration-workshop/stacks ``` - Edit `dockercoins/worker/worker.py`, update the `sleep` line to sleep 1 second - Build a new tag and push it to the registry: ```bash #export REGISTRY=localhost:3xxxx export TAG=v0.2 docker-compose -f dockercoins.yml build docker-compose -f dockercoins.yml push ``` ] .debug[[kube/rollout.md](https://github.com/jpetazzo/container.training/tree/osseu17/slides/kube/rollout.md)] --- ## Rolling out the new version of the `worker` service .exercise[ - Let's monitor what's going on by opening a few terminals, and run: ```bash kubectl get pods -w kubectl get replicasets -w kubectl get deployments -w ``` - Update `worker` either with `kubectl edit`, or by running: ```bash kubectl set image deploy worker worker=$REGISTRY/worker:$TAG ``` ] -- That rollout should be pretty quick. What shows in the web UI? .debug[[kube/rollout.md](https://github.com/jpetazzo/container.training/tree/osseu17/slides/kube/rollout.md)] --- ## Rolling out a boo-boo - What happens if we make a mistake? .exercise[ - Update `worker` by specifying a non-existent image: ```bash export TAG=v0.3 kubectl set image deploy worker worker=$REGISTRY/worker:$TAG ``` - Check what's going on: ```bash kubectl rollout status deploy worker ``` ] -- Our rollout is stuck. However, the app is not dead (just 10% slower). .debug[[kube/rollout.md](https://github.com/jpetazzo/container.training/tree/osseu17/slides/kube/rollout.md)] --- ## Recovering from a bad rollout - We could push some `v0.3` image (the pod retry logic will eventually catch it and the rollout will proceed) - Or we could invoke a manual rollback .exercise[ - Cancel the deployment and wait for the dust to settle down: ```bash kubectl rollout undo deploy worker kubectl rollout status deploy worker ``` ] .debug[[kube/rollout.md](https://github.com/jpetazzo/container.training/tree/osseu17/slides/kube/rollout.md)] --- ## Changing rollout parameters - We want to: - revert to `v0.1` - be conservative on availability (always have desired number of available workers) - be aggressive on rollout speed (update more than one pod at a time) - give some time to our workers to "warm up" before starting more The corresponding changes can be expressed in the following YAML snippet: .small[ ```yaml spec: template: spec: containers: - name: worker image: $REGISTRY/worker:v0.1 strategy: rollingUpdate: maxUnavailable: 0 maxSurge: 3 minReadySeconds: 10 ``` ] .debug[[kube/rollout.md](https://github.com/jpetazzo/container.training/tree/osseu17/slides/kube/rollout.md)] --- ## Applying changes through a YAML patch - We could use `kubectl edit deployment worker` - But we could also use `kubectl patch` with the exact YAML shown before .exercise[ .small[ - Apply all our changes and wait for them to take effect: ```bash kubectl patch deployment worker -p " spec: template: spec: containers: - name: worker image: $REGISTRY/worker:v0.1 strategy: rollingUpdate: maxUnavailable: 0 maxSurge: 3 minReadySeconds: 10 " kubectl rollout status deployment worker ``` ] ] .debug[[kube/rollout.md](https://github.com/jpetazzo/container.training/tree/osseu17/slides/kube/rollout.md)] --- name: toc-next-steps class: title Next steps .nav[[Back to table of contents](#toc-chapter-1)] .debug[(automatically generated title slide)] --- # Next steps *Alright, how do I get started and containerize my apps?* -- Suggested containerization checklist: .checklist[ - write a Dockerfile for one service in one app - write Dockerfiles for the other (buildable) services - write a Compose file for that whole app - make sure that devs are empowered to run the app in containers - set up automated builds of container images from the code repo - set up a CI pipeline using these container images - set up a CD pipeline (for staging/QA) using these images ] And *then* it is time to look at orchestration! .debug[[kube/whatsnext.md](https://github.com/jpetazzo/container.training/tree/osseu17/slides/kube/whatsnext.md)] --- ## Namespaces - Namespaces let you run multiple identical stacks side by side - Two namespaces (e.g. `blue` and `green`) can each have their own `redis` service - Each of the two `redis` services has its own `ClusterIP` - `kube-dns` creates two entries, mapping to these two `ClusterIP` addresses: `redis.blue.svc.cluster.local` and `redis.green.svc.cluster.local` - Pods in the `blue` namespace get a *search suffix* of `blue.svc.cluster.local` - As a result, resolving `redis` from a pod in the `blue` namespace yields the "local" `redis` .warning[This does not provide *isolation*! That would be the job of network policies.] .debug[[kube/whatsnext.md](https://github.com/jpetazzo/container.training/tree/osseu17/slides/kube/whatsnext.md)] --- ## Stateful services (databases etc.) - As a first step, it is wiser to keep stateful services *outside* of the cluster - Exposing them to pods can be done with multiple solutions: - `ExternalName` services
(`redis.blue.svc.cluster.local` will be a `CNAME` record) - `ClusterIP` services with explicit `Endpoints`
(instead of letting Kubernetes generate the endpoints from a selector) - Ambassador services
(application-level proxies that can provide credentials injection and more) .debug[[kube/whatsnext.md](https://github.com/jpetazzo/container.training/tree/osseu17/slides/kube/whatsnext.md)] --- ## Stateful services (second take) - If you really want to host stateful services on Kubernetes, you can look into: - volumes (to carry persistent data) - storage plugins - persistent volume claims (to ask for specific volume characteristics) - stateful sets (pods that are *not* ephemeral) .debug[[kube/whatsnext.md](https://github.com/jpetazzo/container.training/tree/osseu17/slides/kube/whatsnext.md)] --- ## HTTP traffic handling - *Services* are layer 4 constructs - HTTP is a layer 7 protocol - It is handled by *ingresses* (a different resource kind) - *Ingresses* allow: - virtual host routing - session stickiness - URI mapping - and much more! - Check out e.g. [Træfik](https://docs.traefik.io/user-guide/kubernetes/) .debug[[kube/whatsnext.md](https://github.com/jpetazzo/container.training/tree/osseu17/slides/kube/whatsnext.md)] --- ## Logging and metrics - Logging is delegated to the container engine - Metrics are typically handled with Prometheus (Heapster is a popular add-on) .debug[[kube/whatsnext.md](https://github.com/jpetazzo/container.training/tree/osseu17/slides/kube/whatsnext.md)] --- ## Managing the configuration of our applications - Two constructs are particularly useful: secrets and config maps - They allow to expose arbitrary information to our containers - **Avoid** storing configuration in container images (There are some exceptions to that rule, but it's generally a Bad Idea) - **Never** store sensitive information in container images (It's the container equivalent of the password on a post-it note on your screen) .debug[[kube/whatsnext.md](https://github.com/jpetazzo/container.training/tree/osseu17/slides/kube/whatsnext.md)] --- ## Managing stack deployments - The best deployment tool will vary, depending on: - the size and complexity of your stack(s) - how often you change it (i.e. add/remove components) - the size and skills of your team - A few examples: - shell scripts invoking `kubectl` - YAML resources descriptions committed to a repo - [Helm](https://github.com/kubernetes/helm) (~package manager) - [Spinnaker](https://www.spinnaker.io/) (Netflix' CD platform) .debug[[kube/whatsnext.md](https://github.com/jpetazzo/container.training/tree/osseu17/slides/kube/whatsnext.md)] --- ## Cluster federation -- 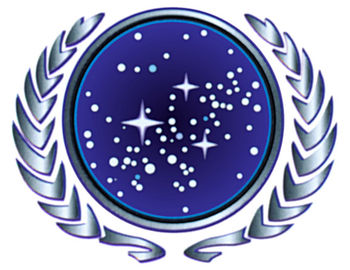 -- Sorry Star Trek fans, this is not the federation you're looking for! -- (If I add "Your cluster is in another federation" I might get a 3rd fandom wincing!) .debug[[kube/whatsnext.md](https://github.com/jpetazzo/container.training/tree/osseu17/slides/kube/whatsnext.md)] --- ## Cluster federation - Kubernetes master operation relies on etcd - etcd uses the Raft protocol - Raft recommends low latency between nodes - What if our cluster spreads multiple regions? -- - Break it down in local clusters - Regroup them in a *cluster federation* - Synchronize resources across clusters - Discover resources across clusters .debug[[kube/whatsnext.md](https://github.com/jpetazzo/container.training/tree/osseu17/slides/kube/whatsnext.md)] --- ## Developer experience *I've put this last, but it's pretty important!* - How do you on-board a new developer? - What do they need to install to get a dev stack? - How does a code change make it from dev to prod? - How does someone add a component to a stack? .debug[[kube/whatsnext.md](https://github.com/jpetazzo/container.training/tree/osseu17/slides/kube/whatsnext.md)] --- class: title That's all folks!
Questions? .small[.small[ Jérôme ([@jpetazzo](https://twitter.com/jpetazzo)) — [@docker](https://twitter.com/docker) ]] .debug[(inline)]